We all know about colorimetric color constancy, which for us in color imaging mostly takes the form of white point estimation and white balancing. The November 12, 2009 issue of the Journal of Vision has an interesting article by Maria Olkkonen et al. on a different kind of color constancy.
Color constancy in general refers to perceived color of objects not changing much when seen under different illuminations. In their paper Categorical color constancy for simulated surfaces, Maria Olkkonen, Thorsten Hansen, and Karl R. Gegenfurtner study the communication of color under different illuminations.
Color communication entails color naming and color naming entails categorical color. The authors start with the reflectance spectra of the Munsell color chips, calculate their tristimulus values under five different illuminations, and display them on a Sony Multiscan GDM-F520 monitor, whose gamut restricts them to limit the chips to 469 samples.
In a very diligently executed psychophysical experiment, four observers categorize each sample into one of the categories green, turquoise, blue, purple, red, orange, yellow, brown, or gray. The categories for orange and brown are pooled.
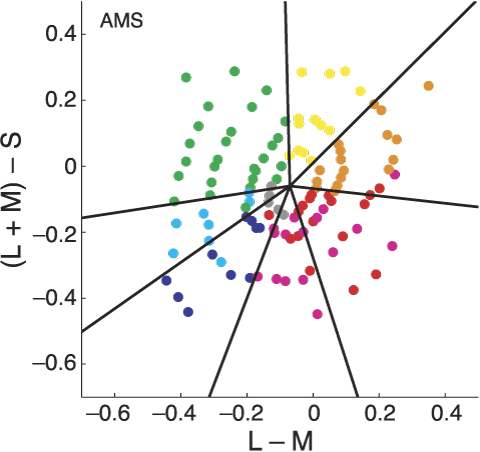
Since color matching is not involved, the authors are not using a colorimetric color space, but a perceptual color space, viz. the DKL color space, which is a linear transformation of the cone excitation space based on the Smith and Pokorny cone fundamentals. DKL is for Derrington, Krauskopf, and Lennie.
The observers perform the color naming experiment twice, with a 6 month hiatus, yielding a measure for the reliability of color naming. With this "calibration" the categorical color constancy is nearly perfect.
This result is important because the World Color Survey is performed under unrecorded random ambient conditions, a fact that has often been criticized. As we reported in our EI paper, Boynton wrote "the mechanisms of color constancy work so well that, within limits, the intensity and spectral distribution of the light used to illuminate the experimental materials make surprisingly little difference," but that was just an intuition. With their painstakingly rigorous experiment, Maria Olkkonen, Thorsten Hansen, and Karl R. Gegenfurtner deliver a definitive proof.
Some points to note:
- In rigorous experiments, you can only have a small number of conforming observers (see Mavericks are best for crowd-sourcing)
- When not doing color matching, a perceptual color space is better than a colorimetric one
- They limit the categories to nine color terms
- Yes Virginia, color naming via crowd-sourcing is legitimate